A/B Testing Guide: The Proven 6-Step Process for Higher Conversions
Welcome to the ultimate guide to A/B testing, a comprehensive resource designed to help you master the art of optimizing your digital experiences. Whether you’re a marketer, a product manager, or a business owner, understanding and implementing A/B testing can be a game-changer for your online presence.
Effective CRO services include A/B testing because they’re the only data-driven method for increasing conversions and powering growth.
Key Takeaways
- Data-Driven Decision Making: A/B testing removes guesswork by providing clear, measurable insights into what works and what doesn’t.
- Continuous Improvement: Optimization is an ongoing process. Each test builds on previous learnings to create a culture of experimentation and innovation.
- Understanding Your Audience: Pre-test research and segmentation help you tailor your tests to specific user behaviors and preferences for more impactful results.
- Statistical Rigor: Achieving statistical significance ensures that your conclusions are reliable and not influenced by chance.
- Collaboration and Communication: Sharing results with stakeholders and aligning them with business goals ensures long-term success.
Table of Contents
- A/B Testing Meaning
- What to Expect from This Guide
- Benefits of A/B Testing
- The Key to A/B Testing Success
- The A/B Testing Process
- Common Challenges in A/B Testing
- Conclusion
A/B Testing Meaning
A/B testing, also known as split testing or A/B/n testing, is the process of comparing two or more versions of a webpage, app, or marketing asset to determine which one performs better. It takes a scientific approach to optimizing digital experiences, removing the biases inherent in decision-making based on personal preference.
A/B testing randomly divides users into two groups, serving each group a different variation (A or B) to determine which gets the best results — essentially letting your visitors tell you what they prefer.
What to Expect from This Guide
This guide is designed to be your go-to resource for everything related to A/B testing. Here’s what you’ll learn:
- The A/B Testing Process: Step-by-step guidance on conducting pre-test research, setting up tests, ensuring quality assurance, and analyzing results.
- Common A/B Testing Strategies: Discover various testing strategies such as Gum Trampoline, Completion Optimization, Flow Optimization, and more.
- Tips on Implementing Test Results: How to implement winning variations and document your findings for future improvements.
- Common Challenges with A/B Testing: Tips on handling timing issues, visitor segmentation, and achieving statistical significance.
By the end of this guide, you will have a thorough understanding of the entire A/B testing process and a framework for running your own A/B tests. Whether you are just starting out or upleveling your game, this guide will help you optimize your website and drive business growth through data-backed decisions.
Need help right away? Schedule a Strategy Session with the Conversion Scientists.™
Benefits of A/B Testing
A/B testing can significantly improve your online presence and drive business growth. Here are some of the key advantages of incorporating A/B testing into your optimization strategy:
Improved Performance and Engagement
- Increased Conversion Rates: By comparing different versions of a web page, you can identify which page elements lead to higher conversions.
- Reduced Bounce Rates: Testing helps optimize content and design to keep users engaged and on-site longer.
- Enhanced User Experience: Through iterative testing, you can tailor your digital assets to user preferences, resulting in more satisfying interactions.
Improved Performance and Engagement
- Quantitative Insights: A/B tests yield clear, measurable results that can be easily analyzed and presented to stakeholders.
- Risk Minimization: By testing changes before full implementation, you can avoid making page or website updates that could have a negative impact on key metrics.
- Informed Product Development: Test results can guide future product roadmaps and feature prioritization.
Business Growth and Innovation
- Increased Revenue: Optimizing user experiences through A/B testing often leads to higher sales and improved return on investment (ROI). Even small improvements in conversion rates can translate into significant revenue increases over time.
- Competitive Advantage: A culture of continuous testing and improvement can set your business apart from competitors who rely on guesswork.
- Deeper Audience Insights: A/B tests reveal valuable information about user behavior and preferences, enabling more targeted marketing strategies.
Operational Efficiency
- Traffic Optimization: As traffic becomes more expensive, the rate at which online businesses are able to convert incoming visitors becomes more critical. A/B testing helps in optimizing this conversion rate, ensuring that you get the most out of your traffic.
- Incremental Improvements: A/B testing allows you to make small improvements that are more cost-effective than major overhauls.
- Continuous Improvement: Regular testing enables ongoing refinement of your digital assets and marketing strategies. This continuous cycle of testing and improvement ensures that your website or app remains optimized and aligned with user needs over time.
By leveraging A/B testing, you can make informed decisions, improve user experiences, and ultimately drive business growth through data-backed optimizations. This scientific approach to optimization empowers you to move beyond guesswork and subjective opinions, ensuring that your digital strategies are always aligned with user needs and market trends.
The Key to A/B Testing Success
To enjoy the benefits of A/B testing, we need to be confident that our results are valid and can be used to make informed decisions. That’s where statistical significance comes in.
Statistical significance measures the possibility that the observed difference between two variations (A and B) is due to chance rather than a real effect. It is typically expressed as a probability or p-value. When the p-value is below 0.05, it means there is less than a 5% chance that the observed difference occurred randomly.
In practical terms, statistical significance confirms that the changes made in the winning variation do, in fact, perform better than the losing variation and are not random fluctuations in the data. That’s why we always run a test until statistical significance is achieved.
It’s tempting to end a test when one variation begins to outperform the other, but test results will fluctuate through the test. Only when statistical relevance has been achieved can you be sure of the winner. That’s why most A/B testing platforms have built-in calculators. If not, you can find them online — here’s one by VWO.
Keep in mind, statistical significance does not indicate the impact or the practical importance of the change you’re testing. It simply gives you 95% confidence that the test is valid.
Now, Let’s dive into the A/B testing process. We’ll start with a high-level look at the steps to A/B testing and then review each of each step.
The A/B Testing Process: Step-by-Step Guide
The A/B testing process is a systematic and methodical approach to optimizing digital experiences. Here’s the six-step testing process used by CRO professionals:
Here’s the six-step testing process used by CRO professionals:
Step 1: Conduct Pre-test Research. A/B testing starts with assessing your current data. Gather both quantitative data (numbers and metrics) and qualitative data (user feedback and behavior) to gain a comprehensive understanding of your website’s performance and user experience.
Jump to: How to perform pre-test research
Step 2: Generate Hypotheses. Based on your data analysis, create hypotheses about what changes might improve your website’s performance. This step marks the beginning of experimentation, where you identify potential page improvements to test.
Jump to: How to generate a hypothesis
Step 3: Design A/B Split Tests. Create your control (the original, or A version) and variation (the B version) based on your hypotheses. Ensure that you’re testing one variable at a time to isolate its impact.
Jump to: How to design A/B split tests
Step 4: Run and Monitor Tests. Use A/B testing software to split your traffic between variations until statistical significance is achieved. Monitor the test closely to ensure data accuracy and catch any potential issues early.
Jump to: How to run and monitor tests
Step 5: Analyze Test Results. Analyze your test results for statistical significance. Look beyond just the overall results to understand how different segments performed.
Jump to: How to evaluate test results
Step 6: Implement Results. If you have a clear winner, implement the changes across your website. Continue to monitor performance to ensure the improvements are maintained over time.
Jump to: How to implement results
Now let’s explore each of these steps more deeply:
Step 1: Pre-Test Research
Pre-test research is the process of gathering and analyzing data to understand your visitors, their behaviors, and their needs before you start designing test variations.
This step is key because, at its core, optimization is about understanding your visitors. To create effective A/B test variations, you need to know who is visiting your website, what they like and don’t like about your existing site, and what they want instead. This insight allows you to formulate hypotheses that have a higher likelihood of improving user experience and driving conversions.
Steps to Conduct Pre-Test Research
Gather Existing Data. Start by analyzing the data you already have. Use tools like Google Analytics, heatmaps, and session recordings to understand user behavior on your website. Look for patterns in:
- Traffic sources: Where are your visitors coming from?
- Bounce rates: Which pages are causing visitors to leave quickly?
- Conversion rates: Which pages or elements are performing well or poorly?
- User flow: How are visitors navigating your site?
For example, you might discover that visitors from social media platforms have a higher bounce rate on your product pages compared to visitors who click through from search engines. This insight could lead to a hypothesis about tailoring product page content for different traffic sources.
Conduct User Research. Quantitative data tells you what is happening, but qualitative research helps you understand why. These methods can help you gather input from your visitors:
- Surveys: Ask visitors about their experience on your site.
- Feedback forms: Collect specific comments about certain pages or features.
- Usability testing: Observe how real users interact with your site in real time.
For instance, a survey might reveal that customers find your checkout process confusing, leading your team to a hypothesis about simplifying the checkout flow.
Analyze Competitor Data. Study your competitors’ websites to benchmark your own performance and set realistic goals. This can inspire new ideas and opportunities for testing. Look at their:
- Design and layout
- Messaging and value propositions
- Features and functionality
This analysis might show that competitors are using trust badges prominently in their checkout process, suggesting a test idea for your own site.
Identify Key Metrics and Goals. Define the specific metrics you want to improve through A/B testing. For example, for an ecommerce site, you might aim to improve:
- Conversion rate
- Average order value
- Revenue per visit
- Click-through rate
Ensure these metrics align with your overall business objectives. If your goal is to increase customer lifetime value, you might focus on metrics related to repeat purchases or subscription sign-ups.
Segment Your Audience. Identify different user segments that behave differently or have different needs, such as:
- New vs. returning visitors
- Mobile vs. desktop users
- Customers vs. strangers
- Traffic source
Understanding these segments allows you to create more targeted tests and analyze results more effectively. For instance, you might find that a certain call-to-action performs better for new visitors but worse for returning customers.
Step 2: Hypothesis Generation
Once you’ve completed your pre-test research, it time to formulate educated guesses, or hypotheses, about what changes might improve your website’s performance. This is where data-driven insights meet creative problem-solving.
What Is a Hypothesis in A/B Testing?
In A/B testing, a hypothesis is a testable idea. It’s typically structured as an if-then statement that includes a proposed change, the predicted outcome if the change is implemented, and how success will be measured:
“If ___, then ___, as measured by ___.”
For example: “If we move the form above the fold, then sign-ups will increase by 10%, as measured by on-page form completions.”
For more examples, read How to Create Testing Hypotheses That Drive Real Profits
The Hypothesis Generation Process
When creating a hypothesis, it’s a good idea to follow this process:
- Identify Problems and Opportunities. Pinpoint specific issues that need addressing or areas with potential for improvement.
- Analyze Data. Thoroughly review data related to the issue. Look for patterns, anomalies, and areas of underperformance.
- Brainstorm Solutions. For each problem or opportunity, brainstorm potential solutions. Think outside the box and collect a wide range of ideas.
- Formulate Hypotheses. Turn your proposed solutions into structured hypotheses.
- Prioritize. Not all hypotheses are created equal. Each hypothesis should be prioritized according to its potential impact, ease of implementation, and available resources.
Note: While hypothesis creation is listed as step #4 above, in many scenarios, hypotheses are developed as part of the step #1. Data is then collected to better understand the problem and potential solutions. When this approach is taken, you may uncover data or possible solutions that weren’t considered in step #1, invalidating the original hypothesis. When this happens, you can revise and reprioritize the original hypothesis based on the new data.
Tips for Effective Hypothesis Generation
It’s easy to generate hypotheses. The challenge is to create good hypotheses. Here’s how you can ensure your hypothesis pipeline is filled with test-worthy ideas.
- Focus on One Change. A test can be designed to test multiple hypotheses, but each hypothesis should be specific and unambiguous.
- Be Specific. Clearly state the change you’re making and the outcome you expect.
- Make it Measurable. Ensure your predicted outcome can be quantified and measured.
- Base it on Data. Your hypothesis should be grounded in research and data analysis, not just hunches.
- Keep it Realistic. Keep your predictions within the realm of possibility.
- Consider Multiple Factors. Remember that user behavior is complex. Consider various aspects like user psychology, design principles, and industry best practices when forming your hypotheses.
- Document Your Reasoning. Always include the “because” part of your hypothesis. This helps you track your thought process and can inform future tests.
Get the Conversion Sciences Hypothesis Tracker here.
Step 3: A/B Split Test Design
An A/B test is often called a “split” test because it involves splitting or dividing website traffic between two versions of a webpage or feature. The term “split” refers to how the audience or traffic is divided between the control version (A) and the variation (B).
A/B split testing is most effective when you know how to design the test to achieve your goals. This includes your testing strategy, objectives, the variables you’ll test, your segmentation plan, and the set-up of your tracking.
In a minute, I’ll show you how to design a split test, but first, we need to look at seven common A/B testing strategies.
Common A/B Testing Strategies
An A/B testing strategy is a systematic approach to planning, executing, and analyzing experiments. At Conversion Sciences, we use a variety of strategies, each appropriate for different situations.
Will you test big, disruptive changes to the webpage or make small, incremental tweaks? Will you optimize for general outcomes, such as completion rate or flow, or target specific pain points that create friction for visitors? Your strategy choice is based on risk tolerance, the current performance of the website, the resources available, and your specific goals.
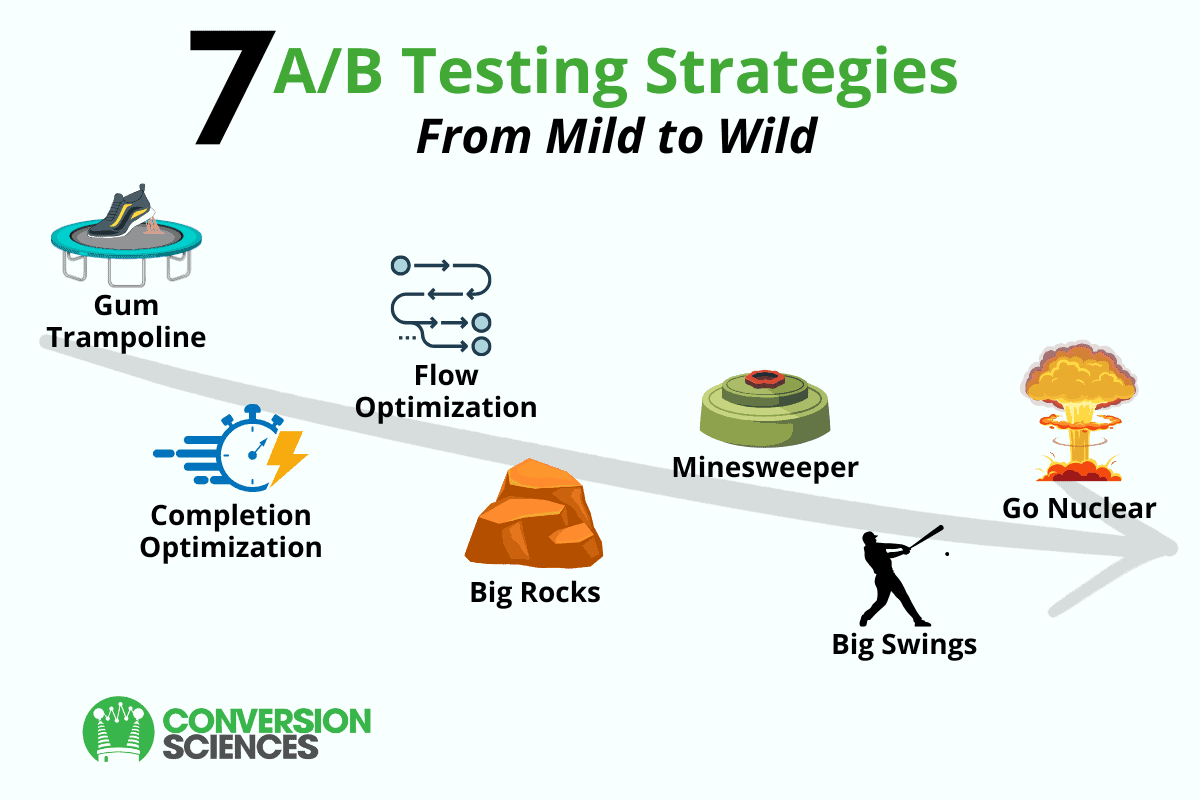
Most optimizers choose one of these seven testing strategies.
Gum Trampoline
- What: Focus on small, incremental changes to improve user experience and performance.
- When: When bounce rates are high, especially from new visitors, or when you need to make continuous, minor adjustments.
- How: Implement small, iterative tests on elements like button colors, font sizes, or minor layout adjustments to accumulate incremental improvements over time.
Completion Optimization
- What: Improving the completion rate of a specific process or user journey.
- When: When a high percentage of people are abandoning a checkout process, lead generation form, or registration form.
- How: Improve the completion rate of that action by testing variations such as simplified forms, clearer instructions, or reduced steps in the process.
Flow Optimization
- What: Enhancing the user journey or flow through a website, app, or any digital experience.
- When: When you notice high drop-off rates or friction points in the user journey.
- How: Streamline processes, improve navigation and usability, and test different layouts to make the user experience more seamless and efficient.
Minesweeper
- What: Identifying and fixing pain points in the user journey.
- When: When things are broken all over the site, or when there are multiple areas of friction that need immediate attention.
- How: Use analytics and user feedback to identify critical pain points and then test fixes for these issues to improve overall user satisfaction and reduce drop-offs.
Big Rocks
- What: Addressing significant issues that have a large impact on user experience and conversion rates.
- When: Your site has a long history of optimization and ample evidence that an important component is missing or underperforming.
- How: Identify key areas that, when improved, can have a substantial impact on performance. Test significant changes such as new features, major layout overhauls, or critical functionality improvements.
Big Swings
- What: Testing more radical changes to see substantial improvements.
- When: You are looking for significant improvements and are willing to take calculated risks. This could be during periods of low traffic or when you have a robust testing infrastructure in place.
- How: Implement bold, innovative changes such as completely new designs, alternative user flows, or experimental features to see if they can drive substantial improvements in key metrics.
Go Nuclear
- What: Making drastic, fundamental changes to the digital experience.
- When: When you’re changing the backend platform, rebranding the company or product, or undergoing a major redesign.
- How: Conduct thorough A/B testing before and after the major change to ensure the new version performs better than the old one. This involves extensive planning, testing of critical components, and careful analysis to mitigate risks and maximize benefits.
Dive more deeply into the 7 core testing strategies essential to optimization.
How to Design an A/B Split Test: 6 Steps
Effective A/B testing follows a structured, scientific approach to ensure objectivity and validity. Here’s how to set up a robust A/B test:
Choose Your A/B Testing Tool. There are several A/B testing platforms on the market, each with its own strengths, so choose one that aligns with your specific needs and technical capabilities. Here are three popular options:
- Optimizely: A robust platform offering advanced features and integrations, ideal for larger enterprises with complex testing needs.
- VWO: Known for its user-friendly interface and comprehensive features, VWO is suitable for businesses of all sizes.
- AB Tasty: Combines A/B testing with personalization features, making it a good choice for businesses focusing on tailored user experiences.
Note: Read pro optimizers’ recommendations for A/B testing platforms here.
Define Clear Objectives. Start by clearly defining your test goals and hypotheses. Your goals should be specific, measurable, and aligned with your overall business objectives. For example:
- Goal: Increase newsletter sign-ups by 20%
- Hypothesis: Changing the CTA button color from blue to green will increase sign-ups by 10%, as measured by form completions because people associate green with “go.”
Note: Your objectives will inform the type of A/B test you choose from the seven options we reviewed above.
Choose Variables to Test. Select one primary variable to change in your variation. Common test elements include:
- Headlines or copy
- Call-to-action buttons (color, text, placement)
- Images or videos
- Page layout
- Form fields
Create Variations. Design your control (original version) and variation(s) based on your hypothesis. Remember to change only one element to isolate its impact.
Determine Sample Size and Test Duration. Calculate the required sample size to achieve statistical significance. Your testing tool likely has a calculator, but you can also use this one. Keep in mind, your sample size depends on several factors:
- Your current conversion rate: Lower conversion rates usually require larger sample sizes to detect significant changes
- The minimum detectable effect (MDE): This is the smallest improvement you want to be able to detect. Smaller effects require larger sample sizes.
- Statistical significance level: To achieve at least 95% confidence that the results are not due to chance, you’ll need larger sample sizes.
- Statistical power: Usually set at 80%, this is the probability of detecting a real effect. Higher statistical power requires larger sample sizes.
- Population size: For smaller target populations, you’ll need a larger sample size to ensure representativeness. (If you don’t have enough traffic, we don’t recommend A/B tests. Listen to this episode of the Two Guys on Your Website podcast to learn conversion optimization techniques that work for small populations.)
Plan for Segmentation. Consider how you’ll analyze results for different user segments (e.g., new vs. returning visitors, mobile vs. desktop users).
Set Up Tracking. Accurate tracking ensures meaningful results. Here are the key tracking elements that need to be in place:
- Analytics: Set up Google Analytics event tracking for key user actions, and configure goals and conversions relevant to your test objectives.
- A/B testing tool integration: Make sure your chosen testing platform is connected to Google Analytics, your content management system (CMS) and customer relationship management (CRM) systems, and any development tools you use.
- Data validation: Before launching your test, perform an A/A test to validate your tracking setup is working correctly and not introducing any bias or errors.
If prioritizing hypotheses and designing tests seems challenging, consider outsourcing it to the CRO experts at Conversion Sciences.
Step 4: Running an A/B Test
Once you’ve designed your A/B test, you’re ready to set up and run your A/B test. This process involves careful planning, execution, and monitoring to ensure reliable results.
- Create the test versions. The original page is your control (Version A). Create a variation (Version B) to test your hypothesis.
- Set up the test in A/B testing software. The software will split your traffic between these versions. In most cases, each page gets 50% of the traffic, but you can set the percentages in the software.
- Run the test until you reach statistical relevance. Most tests run for at least two weeks, but it may go longer if you haven’t reached statistical relevance yet.
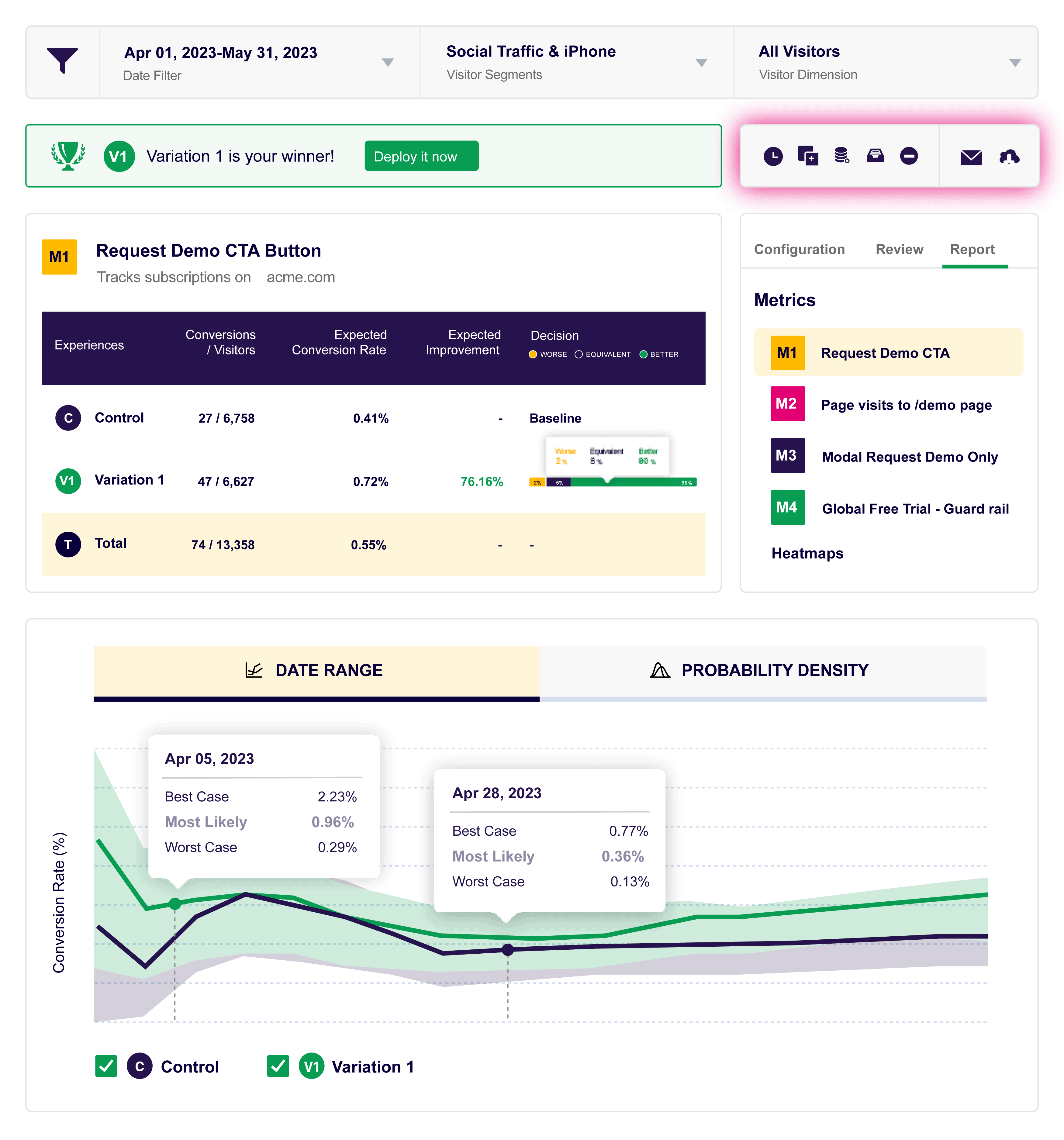
AB testing software VWO test report showing the winning variation.
How to Ensure Quality Assurance
Quality assurance (QA) safeguards the integrity of your experiments and the reliability of your results, minimizing errors and data discrepancies. These QA processes address the technical user experience (UX) and data integrity aspects of a successful A/B test:
- Pre-test Validation. Before launching a test, thoroughly check both the control and variation(s) across different devices, browsers, and operating systems to ensure they display and function correctly.
- Consistent User Experience. Verify that users have a consistent experience regardless of which version they see. This includes checking that all links work, forms submit properly, and there are no visual glitches.
- Data Accuracy and Tracking. Data is key to valid A/B tests. Double-check that your analytics tools are correctly set up to track the metrics you’re testing.
- Fallback and Error Handling. Prepare for potential technical issues by implementing fallback options. If the variation fails to load, ensure users still see the control version to avoid lost conversions.
- Continuous Monitoring. Once the test is live, continuously monitor its performance. Look for any anomalies in the data or unexpected user behavior that might indicate a problem with the test setup.
Step 5: Test Results Analysis
Proper analysis ensures that you draw accurate conclusions and make data-driven decisions. Here’s a comprehensive guide on how to evaluate your A/B test results:
Statistical Significance. The first and most crucial aspect of evaluating test results is determining whether you reached statistical significance. This indicates whether the observed differences between variations are likely due to chance or represent a real effect.
- Confidence Level: Typically, a 95% confidence level is used in A/B testing. This means there’s only a 5% chance that the observed difference is due to random variation.
- P-value: Look for a p-value of less than 0.05, which corresponds to the 95% confidence level. A lower p-value indicates stronger evidence against the null hypothesis.
- Sample Size: Ensure your sample size is large enough to detect meaningful differences. Smaller sample sizes can lead to inconclusive or misleading results.
Note: Take a deep dive into statistical significance in this guide on statistical hypothesis testing.
Practical Significance. While statistical significance tells you if results are reliable, practical significance determines if the change is worth implementing.
- Effect Size: Consider the magnitude of the improvement. A 0.5% increase might be statistically significant but may not justify the effort of implementation.
- Business Impact: Translate the percentage improvement into actual revenue or other key business metrics to assess the real-world impact.
Segment Analysis. Don’t just look at overall results. Analyze how different segments performed:
- Device Types: Compare results across desktop, mobile, and tablet users.
- Traffic Sources: Examine how organic, paid, and direct traffic responded to changes.
- New vs. Returning Visitors: These groups often behave differently and may respond differently to variations.
- Geographic Locations: If applicable, look at performance across different regions.
Secondary Metrics. While focusing on your primary conversion goal, it’s also important to evaluate secondary metrics:
- Engagement Metrics: Time on page, bounce rate, pages per session.
- Micro-conversions: Newsletter signups, add-to-carts, or other steps in the funnel.
- Long-term Metrics: Customer lifetime value, retention rates (if data is available).
Validity Checks. Ensure your test results are valid:
- Test Duration: Confirm the test ran for an appropriate length of time, typically at least two weeks and covering full business cycles.
- External Factors: Consider any external events (e.g., marketing campaigns, seasonality) that might have influenced results.
- Technical Issues: Verify there were no technical problems during the test that could have skewed results.
Analyzing Inconclusive Results. If your test is inconclusive:
- Review Segments: Look for any segments where there were significant differences.
- Power Analysis: Determine if you need a larger sample size to detect an effect.
- Refine Hypothesis: Consider if your initial hypothesis needs adjustment based on the data collected.
Documentation and Learning. Regardless of the outcome:
- Document Findings: Record all aspects of the test, including hypothesis, variations, results, and insights gained.
- Share Insights: Communicate results with stakeholders, explaining both what happened and potential reasons why.
- Iterate: Use learnings to inform future test ideas and hypotheses.
By thoroughly evaluating your A/B test results using these methods, you can ensure that your optimization efforts are based on solid data and insights, leading to more effective improvements in your digital experiences.
Step 6: Implementing Test Results
After conducting a successful A/B test, the next crucial step is to document the results, update stakeholders, and, if you have a winner, implement it effectively.
Documenting and Learning
Proper documentation of your A/B tests builds institutional knowledge and informs future optimization efforts. That’s why optimizers always record test results and insights, including:
- Background and context
- Problem statement and hypothesis
- Test setup and methodology
- Results and analysis
- Insights gained, including any unexpected findings
Documentation should be comprehensive yet concise. We try to strike a balance between providing essential information and avoiding unnecessary details. Use spreadsheets to prioritize test ideas, track experiments, and calculate test results. Docs are preferred for an in-depth analysis of each test — they allow you to capture your insights and learnings and share them with stakeholders.
Insights from previous tests will fuel future optimization efforts. Consider creating a testing roadmap that builds on your learnings. For instance, if highlighting reviews was successful, your next test might explore different ways of presenting these reviews or testing review-based elements on other pages of your site.
Updating Decision-Makers
To ensure the long-term success of your optimization program, it’s crucial to communicate your results effectively to stakeholders. Here’s how to do that:
Communicating Results and Plans to Stakeholders. Prepare a clear, concise presentation of your test results, focusing on the metrics that matter most to your business. For example, instead of just reporting a 15% increase in click-through rate, translate this into estimated revenue impact.
Linking Results to Business Goals. Show how your A/B testing program aligns with and contributes to broader business objectives. For instance, if a key company goal is to increase customer lifetime value, demonstrate how your optimizations in the onboarding process are leading to higher retention rates.
Proposing Next Steps. Based on your results and learnings, present a clear plan for future tests and optimizations. This might include a prioritized list of upcoming tests, resource requirements, and projected impacts.In many organizations, only 10% to 20% of experiments generate positive results, with just one in eight A/B tests driving significant change.
As mentioned above, if a winning test won’t drive significant conversion rate improvements, you may opt not to implement the change — it simply isn’t worth the effort. However, when you do implement a change, you need to be sure the improvements you saw in the test translate into real-world performance gains. Here’s how to do that.
Implementing the Winning Version
Once you’ve identified a statistically significant winner in your A/B test, it’s time to implement this version across your entire user base. You can do this in one of two ways:
Option 1: Replacing the Original with the Winning Variant
This step involves updating your website or application to reflect the changes tested in the winning variation. For example, if your test showed that changing a call-to-action button from “Sign Up” to “Start Free Trial” increased conversions by 15%, you would update all instances of this button across your site.
Option 2: Gradual Rollout
In some cases, especially for high-traffic websites or significant changes, it’s wise to implement the winning version gradually. Initially, you would roll out the change to a small percentage of users (say, 10%) and slowly increase the percentage of visitors who see it until it’s fully launched. This approach allows you to monitor for any unexpected issues that might not have shown up during the test.
Monitoring Post-Implementation Performance
After implementing the winning version, continue to monitor performance to be sure the improvements seen during the test phase are maintained in the long term. Make sure you’re tracking key metrics such as conversion rates, engagement levels, and revenue figures.
False positives occur for a variety of reasons. A test might fail to reach statistical relevance or include too many variations. Or maybe it falls prey to the history effect — something in the outside world that affects people’s perception of your test. We’ve seen this happen in holiday seasons, a news event that has captured people’s attention, and even a competitor launching a new product.
If you implement a change and see a drop in site metrics, you need to respond quickly. First, revert to your control page so you can stop the bleeding. Then try to identify the issue:
- Study your post-implementation data to confirm that the drop in metrics was due to the change and not other factors.
- Review the original test to find issues that might have led to a false positive.
- Check for external factors that could have influenced the test: seasonal trends, marketing campaigns, competitor actions, or technical issues.
- Consider running a follow-up test to verify the original findings and explore alternative hypotheses.
By following these steps, you ensure that the insights gained from your A/B tests are effectively implemented, documented, and leveraged for ongoing optimization. This systematic approach not only improves your current performance but also builds a foundation for continuous improvement in your digital experiences.
Common Challenges in A/B Testing
A/B testing is a powerful tool for optimization, but it comes with its own set of challenges. Understanding and addressing these challenges empowers you to conduct effective tests and obtain reliable results.
Timing Issues and External Factors
As mentioned above, we need to be aware of timing issues and external factors that could deliver fast positives or negatives. Here are a few of the external issues that can impact the validity of an A/B test:
Seasonal Variations: User behavior often changes based on seasons, holidays, or specific times of the year. For example, an e-commerce site might see drastically different conversion rates during the holiday shopping season compared to other times of the year.
Market Fluctuations: Economic changes, industry trends, or sudden market shifts can impact user behavior and skew test results. A stock market crash, for instance, could significantly affect conversion rates on a financial services website, and we all experienced the impact of the pandemic and the uncertain economy that followed.
Competitor Actions: User behavior is easily impacted by competitor promotions or new product launches. If a major competitor starts a significant discount campaign during your test, it could affect your results.
Media Events: News cycles, viral content, or major world events can temporarily change user behavior and attention. A breaking news story related to your industry could suddenly increase or decrease engagement with your site.
Technical Issues: Unexpected technical problems, such as server downtime or slow loading speeds, can impact user experience and skew test results if they occur unevenly across variations.
Marketing Campaign Changes: Changes in your own marketing efforts, such as starting or stopping an ad campaign, can lead to different types of traffic arriving at your site, potentially affecting test results.
Timing issues can potentially lead to false positives or negatives, but in most cases, A/B testing mitigates them by simultaneously testing our variations. In an A/A test, we compare changes made at different times. Different external conditions exist in each test period. A/B testing ensures both the control and treatment groups are exposed to the same external factors during the testing period, allowing you to isolate the impact of the elements you’re testing.
Visitor Segmentation
Another significant challenge in A/B testing is ensuring your results accurately represent your entire audience. This challenge results from the complexity and diversity of user behaviors across different segments of your audience. For example:
Audience Diversity. Your website attracts a wide variety of visitors. Some are tire kickers, students, or researchers. Only a percentage are potential customers, and even these are not a homogeneous group. They can vary significantly based on factors such as:
- Demographics (age, gender, location)
- Device types (desktop, mobile, tablet)
- Traffic sources (organic search, paid ads, social media, email)
- User intent (browsing, researching, ready to purchase)
- New vs. returning visitors
Each of these segments may interact with your website differently and respond to changes in unique ways.
Sample Representation. When conducting an A/B test, you’re typically working with a sample of your overall audience. It’s challenging to ensure the test sample accurately represents your entire user base. If your sample is skewed towards a particular segment, your test results may not apply to your broader audience.
Time-based Variations. User behavior can vary based on time of day, day of the week, or season. For example, B2B websites might see different behaviors during business hours compared to evenings or weekends. Ecommerce sites often experience seasonal fluctuations. These temporal variations can impact test results if not properly accounted for.
Geographic and Cultural Differences. For websites with a global audience, cultural differences and regional preferences can significantly impact user behavior. A change that resonates with users in one country might not have the same effect in another.
Here’s how to ensure your A/B tests accurately represent your entire audience:
- Use proper segmentation in your analysis to understand how different user groups respond to changes.
- Run tests long enough to capture a representative sample of your audience.
- Consider running separate tests for significantly different audience segments.
- Use stratified sampling techniques to ensure all important segments are proportionally represented in your test.
Here are some common ways to segment your traffic:
- Traffic source (e.g., organic search, paid ads, social media, email campaigns, direct)
- Device type (desktop, mobile, tablet)
- New vs. returning visitors
- Geographic location
- Time of day or day of the week
- Customer lifecycle stage (e.g., first-time visitor, repeat customer, loyal customer)
- Referring websites
- Landing pages
- User demographics (age, gender, income level, etc.)
- Browser type
- Operating system
By analyzing these segments separately, you can uncover valuable insights about how different groups interact with your site and tailor your optimization efforts accordingly.
Creating a Culture of Testing
For more than a decade, we’ve advocated for a culture of optimization — equipping everyone to optimize their results through experimentation and A/B testing — because the data it provides can quickly scale growth and profits.
It’s easy to fall into the trap of limiting A/B testing to professional conversion optimizers. But practitioners aren’t the only people capable of running successful tests, especially with today’s technology. The key is to give your team the training, tools and workflows they need.
Booking.com is a good example. For more than ten years, they’ve allowed anyone to test anything, without requiring approval. As a result, they run more than 1,000 rigorous tests at a time, which means at any given time, two visitors will get two different variations of the page.
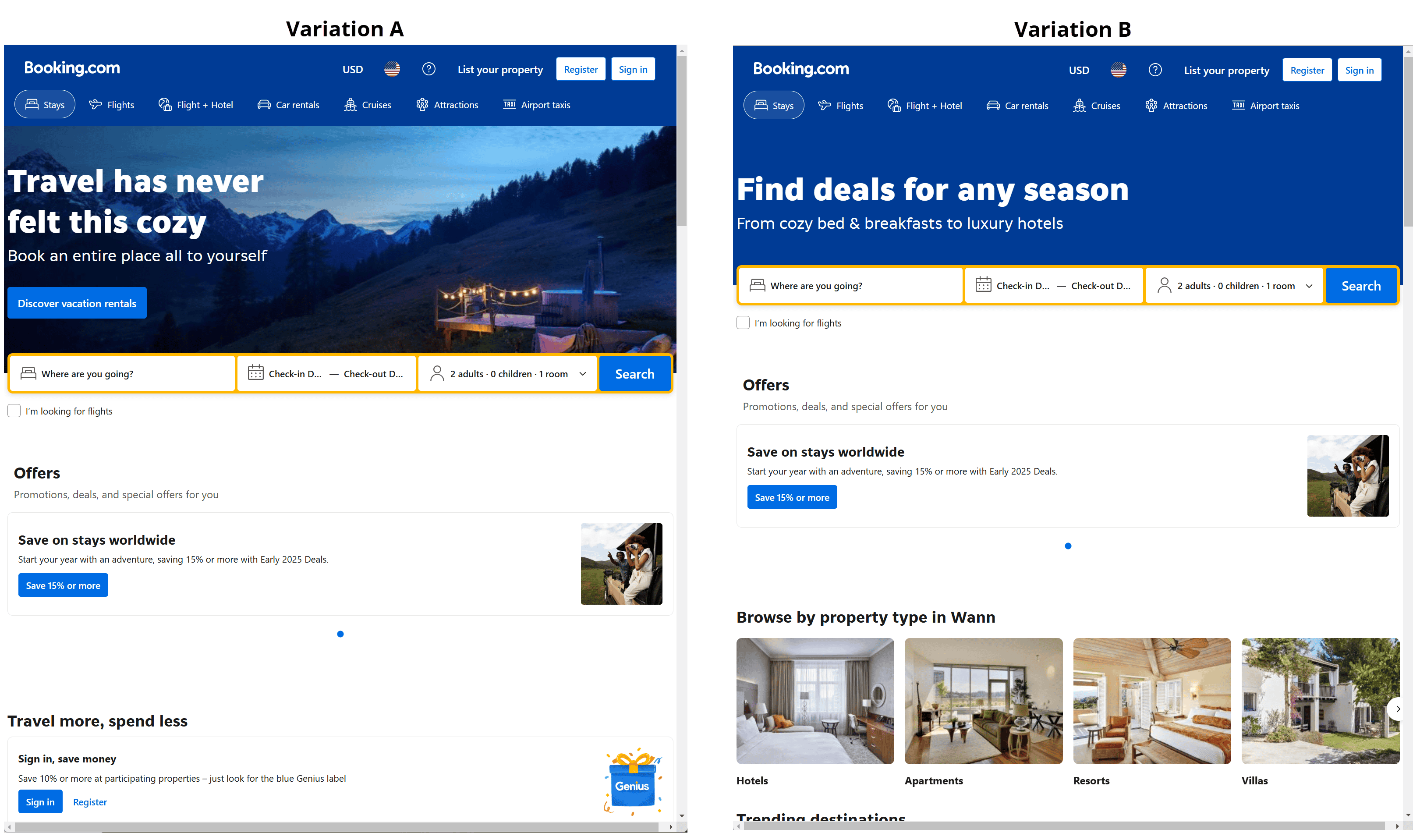
I opened Booking.com from two computers at the same time and got two variations of the page.
Experimentation has become ingrained in Booking.com’s culture, allowing every employee to test their ideas for improved results. Booking.com keeps a repository of tests, both failures and successes, so people can verify the test hasn’t been previously performed.
Conclusion
For optimizers, A/B testing is one of our most powerful strategies for improving website performance, driving conversions, and making data-driven decisions. In this guide, we’ve walked through the entire A/B testing process — from understanding the statistical foundations of hypothesis testing to running tests effectively and overcoming common challenges.
Next Steps
Now that you have a comprehensive understanding of A/B testing, it’s time to put this knowledge into action. Start by identifying areas of your website that could benefit from optimization. Conduct thorough pre-test research, formulate hypotheses based on data, and use the tools and strategies outlined in this guide to run effective tests.
Remember, A/B testing is not a one-time activity — it’s a continuous process. By committing to regular experimentation and learning from every test, you can stay ahead of competitors, delight your users, and drive meaningful business growth.
Whether you’re just starting out or looking to refine your existing program, this guide serves as a roadmap for mastering A/B testing. The journey of optimization is ongoing, but with the right approach, tools, and mindset, the rewards are well worth the effort.
Need help implementing A/B testing on your website? At Conversion Sciences, we have a proven mix of conversion optimization services that can be implemented for sites in every industry.
To learn more, book a conversion strategy session today.
- Statistical Hypothesis Testing: A Simple Guide to Smarter A/B Tests - April 15, 2025
- Four Things You Can Do With an Inconclusive A/B Test - April 14, 2025
- A/B Testing Guide: The Proven 6-Step Process for Higher Conversions - January 29, 2025
I landed on your blog through Growthhackers.com and found out that you guys are doing great. I really loved this A/B test guide. Keep on generating such great content.
Thanks for the kind words!
The portion regarding calculating sample size is incomplete.
You suggest to navigate to this link to calculate if results are significant: https://vwo.com/tools/ab-test-siginficance-calculator/
This calculator is fine to test if the desired confidence interval is met; however, it doesn’t consider whether the correct sample size was evaluated to determine if the results are statistically significant.
For example, let’s use the following inputs:
Number of visitors – Control = 1000, Variant = 1000
Number of conversions – Control = 10, Variant = 25
If plug these numbers into the calculator, we’ll that it met the confidence interval of 95%. The issue is that the sample size needed to detect a lift 1.5% with base conversions of 1% (10 conversions / 1000 visitors) would be nearly 70,000.
Just because a confidence interval was met does NOT mean that sample size is large enough to be statistically significant. This is a huge misunderstanding in the A/B community and needs to be called out.
Colton, you are CORRECT. We would never rely on an AB test with only 35 conversions.
In this case, we can look at the POWER of the test. Here’s another good tool that calculates the power for you.
The example you present shows a 150% increase, a change so significant that it has a power of 97%.
So, as a business owner, can I accept the risk that this is a false positive (<3%) or will I let the test run 700% longer to hit pure statistical significance?